6Convex functions
6.1 Strictly convex functions
Let be a convex subset.
A strictly convex function is a convex function , such that
for every number with and every with .
The strict inequality in
(6.1)
collapses to
an equality if or . For example, if , then the left hand side of
(6.1)
is and
the right hand side is .
Definition
6.1
is illustrated below for a
function . Here both and are real numbers
(that is, in in Definition
6.1
).
The (red) line segment between and lies strictly () above
the (black) graph of :
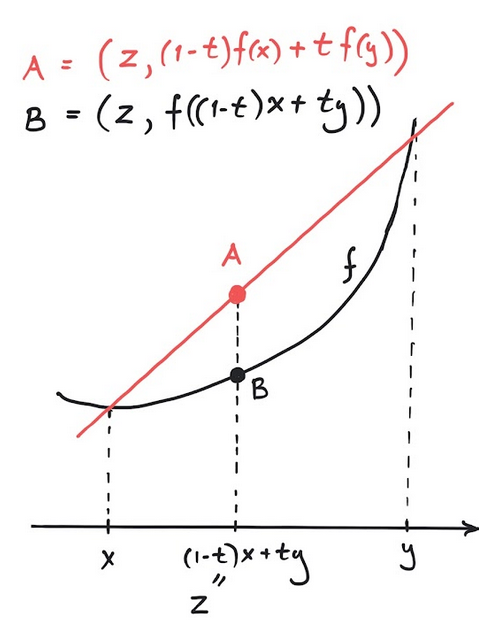
💬
Please explain patiently the definition below to me. It seems that it is also valid
for functions defined on vectors in the plane ($n=2$). Give concrete examples of this.
Test me with a few questions in the end.
'''
Let $C \subseteq \mathbb{R}^n$ be a convex subset.
A \emph{strictly convex function} is a convex function $f: C\rightarrow \mathbb{R}$, such that
\begin{equation}
f((1 - t) u + t v) < (1-t) f(u) + t f(v)
\end{equation}
for every number $t$ with $0< t < 1$ and every $u, v\in C$ with $u\neq v$.
Consider the line (function) given by
for .
This function is convex, since we can formally write for every :
However, the computation in
(6.2)
also shows why
there is no chance that is strictly convex. Intuitively,
the graph of convex functions need to bend and curve a bit to
be strictly convex. No lines should occur in their graphs.
Let be a convex function. Show is strictly convex if and only if
for implies that .
Give an example of a non-constant convex function , which is not strictly convex. Show in
details that is a strictly convex function.
Hint
Look back to the relevant part of Exercise
4.26
for
dealing with .
6.2 Why are convex functions interesting?
A convex function defined on an open convex subset is continuous.
Give an example showing that Theorem
6.7
is not true if the convex function
is defined on a closed convex subset.
Hint
Let be a function, where is an
arbitrary subset (not necessarily convex, open or closed). Then
is called a local minimum for if
for every , which is sufficiently close to . Being
sufficiently close to means that satisfies
for some fixed .
In a much stronger notion, is called a global minimum if
for every (not just locally).
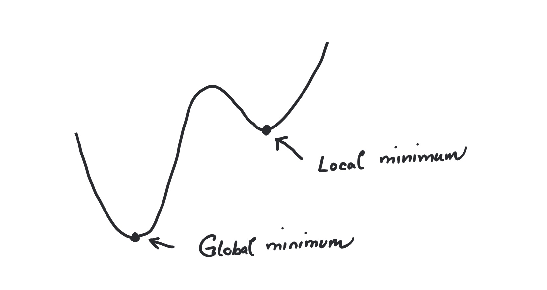
Give an example of a local minimum that is not a global minimum for a precisely specified function.
Also give
an example of a global minimum, which is not uniquely defined (again for a precisely specified function).
Uniquely defined means that there is precisely one , such that is minimal.
Reformulate Definition
6.9
in order to define
a local and a global maximum.
Let be a convex function defined on a convex subset . If is a
local minimum, then is a global minimum. If is strictly
convex, then a global minimum for is unique.
By the definition of local minimum in Definition
6.9
, there exists , such that , when and . Suppose that
is not a global minimum. Then there exists with
. Consider the point
where . Then
Since , we can choose sufficiently small such that
implying , since is a local minimum. This
contradicts that for every . Let
be strictly convex and let be a global minimum for . If
, and , then
for . This would contradict that global minimality
of , since for .
Let be a convex function. Then
for .
6.3 Differentiable functions
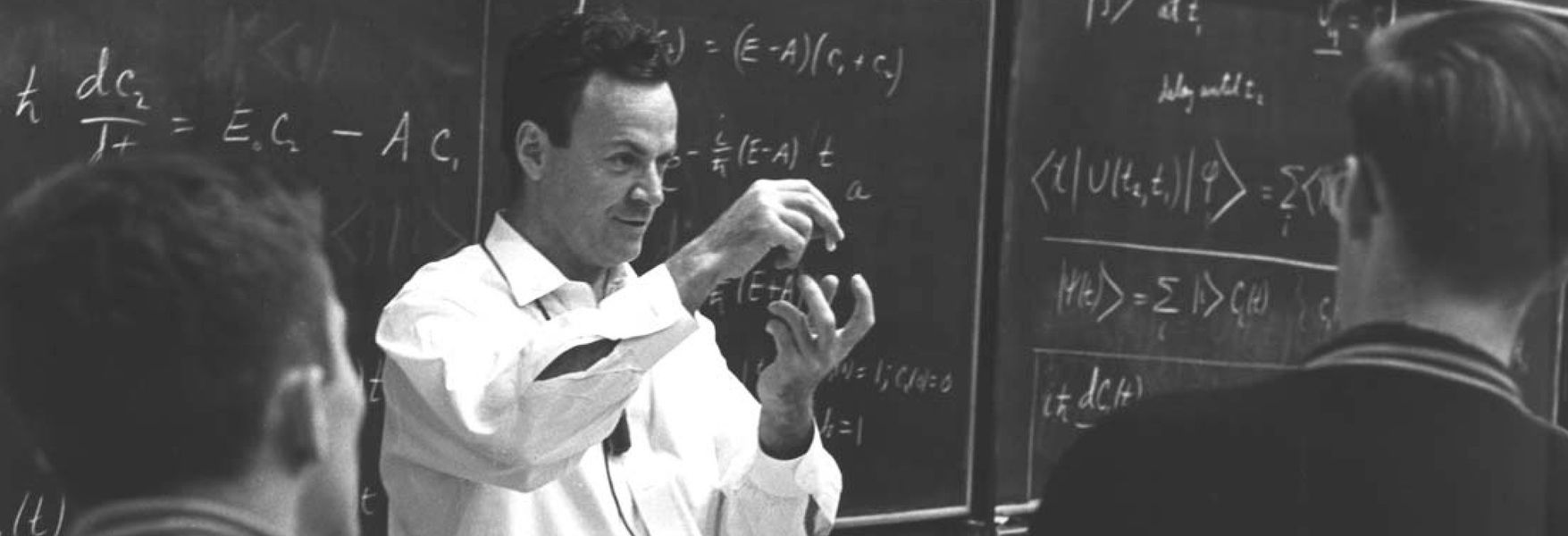
6.3.1 Definition
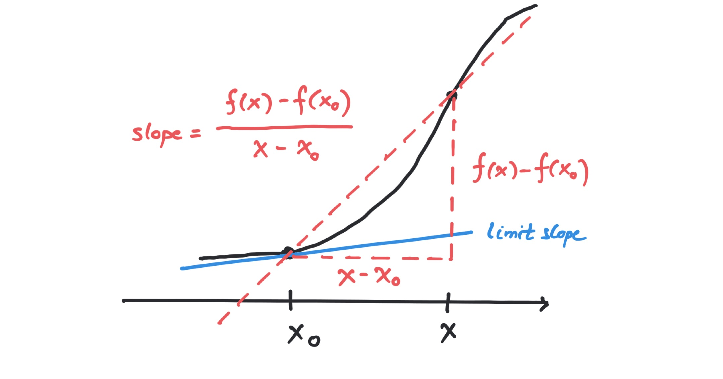
The function is differentiable at if there exists
The number is denoted and called the derivative of
at ; is called differentiable if
it is differentiable at every .
- with i.e., and .
- A function continuous at with ,
💬
Please explain the definition of differentiability given below. Illustrate
by a few example and quiz me afterwards.
'''
The function $f: (a, b)\rightarrow \RR$ is differentiable at $x_0\in (a, b)$ if there exists
\begin{enumerate}[(i)]
\item
$c\in \RR$
\item
$\delta > 0$ with $x_0 - \delta, x_0 + \delta\in (a, b)$ i.e., $a + \delta < x_0$ and $x_0< b-\delta$.
\item
A function $\epsilon: (-\delta, \delta) \rightarrow 0$ continuous at $0$ with $\epsilon(0) = 0$,
\end{enumerate}
such that
\begin{equation}\label{operational}
f(x_0 + h) - f(x_0) = c h + \epsilon(h) h
\end{equation}
for every $h\in (-\delta, \delta)$.
The number $c$ is denoted $f'(x_0)$ and called \emph{the derivative} of
$f$ at $x_0$; $f$ is called \emph{differentiable} if
it is differentiable at every $x_0\in (a, b)$.
'''
If a function is
differentiable, we get a new function
giving the (first) derivative at
a point as output. We may ask again if this function
is differentiable. If this is so, we may define a
function given by
called the second derivative. This
procedure may be continued. We use the notation
for the -th derivative.
Let us apply Definition
6.16
to the function at
the point . Here
Here you immediately see that with
(and ) in Definition
6.16
.
Use Definition
6.16
to formally show that
if .
If the function is differentiable at , then
it is continuous at .
That is continuous at means (recall Definition
5.48
) that to
every , we may find so that
We are
assuming that is differentiable at , so according to Definition
6.16
,
there exists a number so that (with )
I will not write every detail out here, but you can see from the formula above that
for some number , when
is sufficiently small. This gives a that
can be used in
(6.5)
.
The ReLu function is an example of a function, which is
continuous, but
not differentiable at . This is much related to its
sharp corner there.
As mentioned in these notes, the ReLu function plays
a prominent role as an activation function in neural networks.
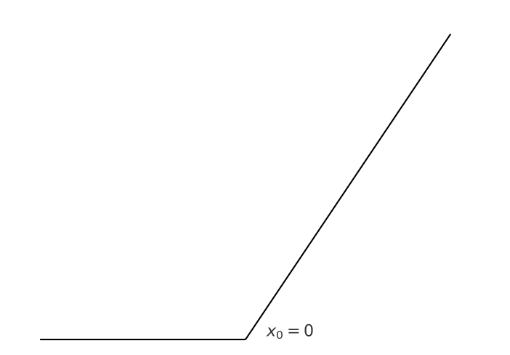
Show precisely that the ReLu function is not differentiable at .
6.3.2 Formulas
- If , where , then .
- If , where , then
- If , then
- If , then Here denotes the logarithm with base .
- If , then
- If , then
- If and are differentiable functions, then the derivative of their product is
- If and are differentiable functions, then the derivative of their quotient is
- If and are composable differentiable functions, then the derivative of their composite is
Suppose that . What is
6.3.3 The derivative of a product
Show how the product rule may be used to derive the rule for finding the derivative of
a fraction:
Hint
6.3.4 The one variable chain rule
For the function for , you already know that . Show that if you define
the function by
for an arbitrary number ,
then .
Compute the derivative of the function given by
using only paper and pencil! You can check your result afterwards using a computer.
Suppose that and are inverse functions i.e.,
If you know the derivative of , how can you use the chain rule
to get the derivative of ? Illustrate with examples like
and , and .
Suppose that is a convex function. We know that
is continuous, but is differentiable at every point ?
Hint
Nope. This is wrong. Come up with a convex function and a point ,
such that is not differentiable at .
6.3.5 The Newton-Raphson method for finding roots
Suppose that and we wish to compute . To do this we may focus on the
quadratic equation and attempt to compute an approximate value ,
such that is close to . Let me at this point disclose that there is
a very effective iterative scheme for doing this. You start by putting and then iterate
using the formula
to get better and better approximations to .
You can try out
(6.11)
below.
The formula in
(6.11)
is derived from
where .
Give an example, where the Newton-Raphson method cycles between points
and never finds the desired zero. Perhaps a drawing will help here.
The formula (see button in Example
1.83
)
for the (monthly) payment on a (car) loan over payments with
a down payment of and an interest rate of (per payment or term) is given by the formula
There is no explicit formula for calculating given and . Here
the Newton-Raphson method is invaluable for estimating by approximating a
zero for the function
Your bank promises you a loan of DKK with yearly payments
of DKK over years. At the same time it claims that
its interest rate is very favorable at only %. Here the bank is
wrong! What is the
real interest rate?
How much money do you save (compared to the original offer from the bank) if you insist that the bank offers you
the promised interest rate of %?
6.3.6 Critical points and extrema
A critical point for a differentiable function is
a point with
Let be a differentiable function. If
is a local extremum for , then is critical point i.e., .
Suppose that is a local maximum and that
according to
(6.4)
. If , then we can choose
sufficiently small, such that if , since and
is continuous in . Therefore
contradicting that is a local maximum. The proof is similar
for and if is a local minimum.
Is the converse of the above lemma true i.e., if is
a local extremum?
Let be continuous and differentiable
on . Then there exists such that
6.3.7 Increasing functions
A function with is called
increasing if
and strictly increasing if
for .
💬
Explain the definition below to me. Give some examples and test me.
\begin{definition}
A function $f:S\rightarrow \mathbb{R}$ with $S\subseteq \mathbb{R}$ is called
\emph{increasing} if
\begin{equation*}
x\leq y\Rightarrow f(x) \leq f(y)
\end{equation*}
and \emph{strictly increasing} if
\begin{equation*}
x< y\Rightarrow f(x) < f(y)
\end{equation*}
for $x, y\in S$.
\end{definition}
Give an example of an increasing function.
Give an example of an increasing function that is not strictly increasing.
Let be a differentiable function.
Then
is increasing if and only if for every . If for every , then is strictly
increasing.
Which of the properties below are true for the function given by
- It is differentiable.
- It is continuous.
- It has a global minimum.
- It has a global maximum.
- It has exactly one critical point.
- It has a local maximum.
- It has a local minimum.
- It is increasing.
- It has three zeros.
- Its derivative has two zeros.
- It is convex.
Show that is strictly increasing i.e.,
Hint
but why is always except when ?
Suppose that is a continuous function, such that
is differentiable on the open interval . Is increasing
on if for every ?
Is it possible for a strictly increasing function to be bounded i.e., does
there exist a (positive) number , such that for every ?
Hint
Have a look at
6.4 Taylor polynomials
Compute the Taylor polynomial for up to degree .
Suppose you have a number that satisfies
Can you make sense of the formula
using Taylor polynomials?
Let be a critical point of an times differentiable
function , such that
is a continuous function,
and . If is even, then is a local
minimum if and a local maximum if
. If is odd, then is not a local extremum.
Let us apply Theorem
6.47
to the function
where . Here and
is a critical point (why?). Since
we see that is a local minimum if and a local maximum if
.
Have you seen Example
6.48
elsewhere, perhaps in a more
geometric setting? What type of curve is the graph of ? Here you may consult
your previous mathematical knowledge.
What is the outcome, when you apply Theorem
6.47
to the
function at ?
Show that is a critical point of the function
defined by
Use Theorem
6.47
in deciding if it is a local
maximum or minimum or neither.
6.5 Differentiable convex functions
Let be a
differentiable function. Then is convex if and only if is
increasing. If is strictly increasing, then is strictly
convex.
Let be a twice differentiable
function. Then is convex if and only if for every
. If for every , then is
strictly convex.
Wait! Stop! Why did I not write if and only if
is strictly convex?
Which of the properties below are true for the function ?
- It is convex on .
- It is strictly convex on .
- It is strictly convex on .
- It is convex on .
- Since , it must have a local minimum for .
You cannot deduce from Corollary
6.52
that the function
given by is a
strictly convex function. Why not?
You can deduce from Corollary
6.52
that is
a strictly convex function. How can
be used to prove that is a strictly convex function?
Show that is a strictly convex function .
Show that is a strictly convex function
.
Show that given by
is a strictly convex function.
Let be a differentiable function. Then
is convex if and only if
for every .
Suppose that is a differentiable
convex function and is a critical point for
. What can you say about using Theorem
6.58
?